Computational cognitive modeling of the predictive active self in situated action (COMPAS)
The COMPAS project aims to develop a computational cognitive model of the Sense of Control (SoC) in situated action. The computational model serves as an explanation of the mechanisms and processes underlying the SoC. It aims at making the following contributions:
- gaining empirical data about the sense of control obtained in experimental studies with human participants
- simulation of situated actions with different sense of controls and analysis of its impact on how actions are regulated with a reinforcement learning agent
Dodge Astroids Scenario
In the scenario, a spaceship has to steer through a world bounded by left and right walls. Different tasks can be done in this scenario, e.g. trying to avoid comets or collect comets. The spaceship is hindered in its actions by noise and drift. The different tasks in the scenario can be solved by humans or computational models. When studying human behavior, the sense of control is measured with questionnaires as well as eye-tracking, and correlated with the observed behavioral strategies to cope with uncertainty in situated actions.
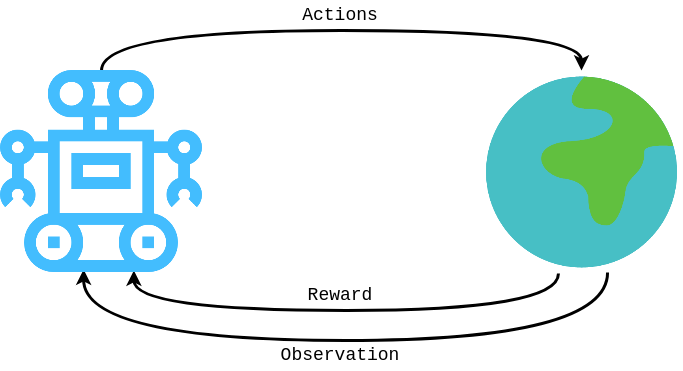
Simulation with a Computational Model
In the COMPAS project, we implement a computational model incorporating a sense of control in situated action. The computational model is based on theoretical processes underlying the sense of control. In our case, a reinforcement learning agent acting in the Dodge Astroids scenario is investigated whether a sense of control can provide control mechanisms shaping the learning of robust and adaptive policies.
Publications
Partner
- U Lübeck : Prof. Nele Russwinkel, Nils Heinrich
- U Bielefeld: Prof. Stefan Kopp, Annika Österdiekhoff
Contact: Annika Österdiekhoff