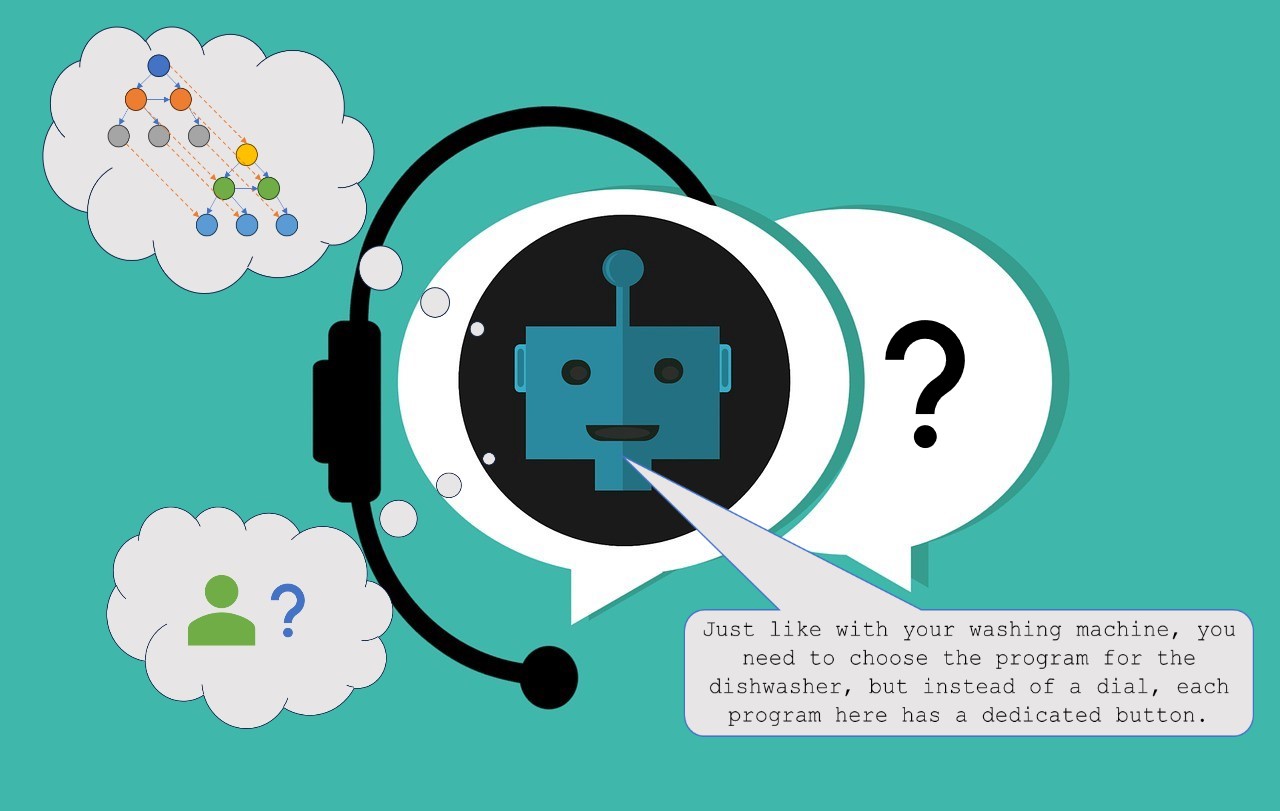
Generating dialog-based analogical explanations about everyday tasks
This project is part of the SAIL network (Sustainable Life-Cycle of Intelligent Socio-Technical Systems) funded by the Ministry of Culture and Science of the State of NRW.
Within SAIL we contribute to the research theme R1: “Human agency to shape cooperative intelligence”. Various tasks and domains exist where humans can benefit from a cooperation with intelligent systems, provided that the system can correctly respond to the needs of the user and the user has the agency to influence the system’s response, for example, by setting goals or providing feedback. We are developing an assistive system with the goal to support users in the accomplishment of everyday tasks at home. The system interacts with the users by means of spoken dialogue and provides analogical explanations that build up on the user’s existing knowledge and enable its transfer to a new domain. By using analogies as a natural means of explanation, we aim to make the system cognitively ergonomic. We are also researching the effects of such a system by evaluating its use under field conditions.
Our approach
We tackle the problem of planning an appropriate analogical explanation based on both (1) domain knowledge and (2) mechanisms of dialog interaction. Both aspects are captured in the system’s conversational memory in terms of a graph-based representation integrating domain knowledge with dialogue state and history information. When trying to explain a new domain to the user, the system applies the structure-mapping theory of human analogical reasoning (by Gentner) in order to identify candidate domains to be the source of an analogy for the current explanandum (the thing being explained). At the same time, the system evaluates these candidates with respect to various constraints imposed by the social nature of adaptive explanations.
Based on observable signals from the explainee (the agent receiving the explanation, in this case the user), the explainer makes predictions about relevant mental states of the explainee, e.g., understanding, interest, engagement, and adapts her behaviour and the presentation of the explanandum accordingly. We are working on mechanisms that allow the system to predict what the user might have inferred from previously established information, how well previous information might have been retained, what kind of user feedback is expected in response to an explanation, etc. These predictions are the constraints for the explanation planning and analogy source selection.
Eventually, the system is supposed to interact with users by means of spoken dialogue. Large language models (LLMs) offer a lot of advantages in natural language processing, e.g., they can be used for language understanding to allow users to speak freely about complex concepts, or they can be used for automated generation of domain knowledge representations from text. However, the operation of LLMs requires significant resources, especially under low-latency conditions for live interaction. We are thus investigating and evaluating the use of smaller LLMs, fine-tuned for specific tasks in our dialogue processing pipeline and will apply our findings by building an LLM-supported dialogue system that can process spoken language in an incremental fashion (based on our dialogue management framework flexdiam).